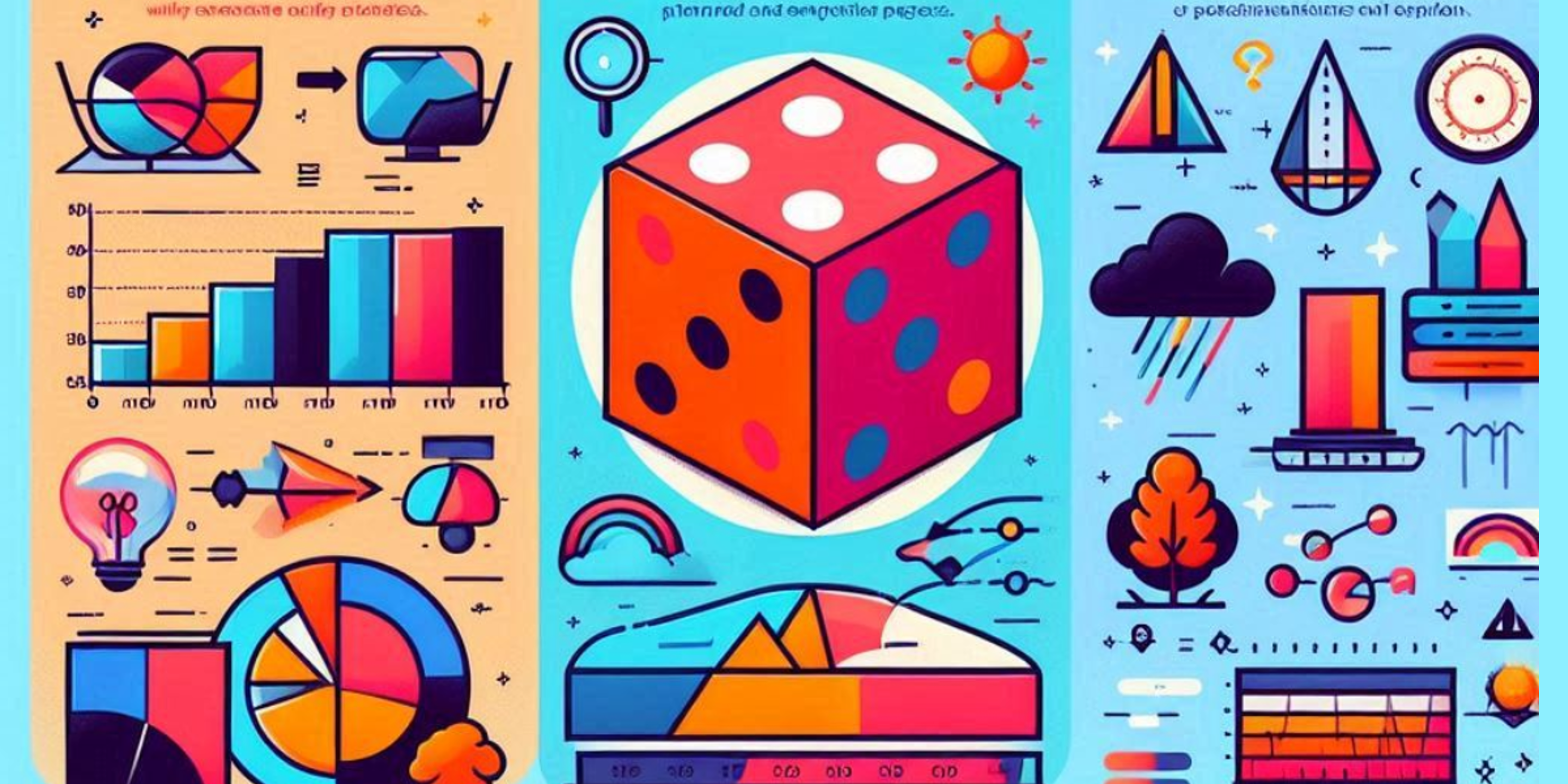
Essential Concepts and Formulas for Business and Economics
In the study of statistics and probability, especially when applied to business and economics, several foundational concepts are essential. These concepts help us make informed decisions under uncertainty by quantifying the likelihood of various outcomes. Let’s explore these key topics, using examples from real-world scenarios, along with their corresponding formulas.
Definitions of Core Probability Terms
Before diving into specific techniques, it’s important to define some fundamental terms. Probability refers to the likelihood of an event occurring, represented as a number between 0 and 1. Experiments are processes that yield outcomes, and each result of an experiment is called an outcome. An event is a set of outcomes of an experiment.
The formula for probability is:
This formula calculates the likelihood of a specific outcome occurring.
Classical, Empirical, and Subjective Probability
Probability can be classified into different types based on how it is calculated. In classical probability, all outcomes are assumed to be equally likely. For example, in a deck of 52 cards, the probability of drawing a king is:
Empirical probability is based on observed data. The formula is:
For instance, if 65 out of 100 surveyed students prefer online learning:
Lastly, subjective probability relies on personal judgment rather than data. For example, if you believe there’s a 70% chance of rain tomorrow based on your interpretation of the weather, this is a subjective probability.
Addition and Complement Rules of Probability
The addition rule allows us to find the probability that one of two events occurs. For events A and B, the formula is:
For instance, if 60% of tourists visit Site A, 50% visit Site B, and 30% visit both, the probability that a tourist visited at least one site is:
The complement rule is used to determine the probability that an event does not occur. The formula is:
If the probability of visiting Site A is 70%, then the probability of not visiting Site A is:
Discrete and Continuous Distributions
Understanding probability distributions is crucial for analyzing different types of data. In a binomial distribution, where events have two possible outcomes (e.g., pass or fail), the formula for finding the probability of exactly successes in independent trials is:
For example, if 80% of products pass quality control and you test 10 products, the probability that exactly 8 pass is:
The Poisson distribution helps model rare events. The formula for the Poisson distribution is:
where is the average rate of occurrence and is the number of expected occurrences.
For example, if a company receives an average of 2 complaints per day, the probability of receiving exactly 3 complaints on a given day is:
Similarly, the exponential distribution models the time between events. The formula is:
where is the rate parameter and is the expected time interval. Rate parameter is calculated as
If the average time between customer arrivals is 10 minutes, the probability that the next customer arrives within 5 minutes is:
The Power of Bayes’ Theorem
In situations involving conditional probabilities, Bayes’ Theorem is a powerful tool. The formula is:
For example, if a factory tests products for defects, with a 5% defect rate, a 95% true positive rate, and a 2% false positive rate, the probability that a product is defective given a positive test result is:
This event can also be represented as probability tree diagram as shown below:
Contingency Tables for Joint Probability
Contingency tables are useful for calculating joint probabilities, such as the likelihood that a randomly selected individual from a group has a specific combination of characteristics. The formula for the joint probability of two events is:
For example, in the following table showing car preferences by gender
Gender | Sedan | SUV | Total |
---|---|---|---|
Male | 40 | 60 | 100 |
Female | 50 | 50 | 100 |
Total | 90 | 110 | 200 |
the probability that a randomly selected person is female and prefers a sedan is:
Probability of selecting someone who prefers an SUV:
This post illustrates the practical applications of probability theory in real-life business scenarios and includes the key formulas you need to solve such problems. Whether dealing with quality control in manufacturing or predicting consumer behavior, a solid understanding of probability enables businesses to operate more effectively under uncertainty.